การรักษา Office Syndrome รามคําแหงเป็นทางเลือกที่ดีสำหรับผู้ที่อยู่ในย่านรามคำแหงหรือบริเวณใกล้เคียงเพื่อสามารถเดินทางมารักษาโรคออฟฟิศซินโดรม (Office Syndrome) ได้อย่างสะดวก เพราะเป็นโรคที่พบได้บ่อยในวัยทำงาน ส่งผลให้มีอาการปวดตามบริเวณต่าง ๆ บางรายมีอาการรุนแรงถึงขั้นปวดเรื้อรังเพราะไม่รู้ว่าป่วยเป็นออฟฟิศซินโดรมจึงปล่อยทิ้งไว้ วันนี้เราจึงจะชวนเพื่อน ๆ ไปตรวจเช็กอาการปวดของตัวเองกันว่าเข้าข่ายโรคออฟฟิศซินโดรมหรือไม่? เพื่อเตรียมรักษาได้ทันเวลา ออฟฟิศซินโดรม (Office Syndrome) คืออะไร อันตรายไหม ? […]
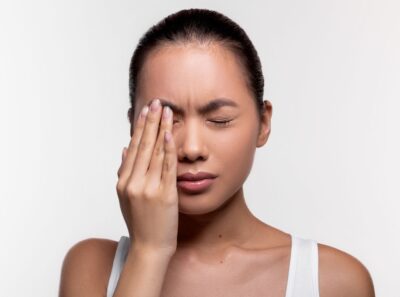
รู้จักกับ meibomian gland dysfunction
เชื่อได้เลยว่าหลายคนคงเคยมีอาการแสบตา สายตาล้าง่าย ไม่สบายตา มองภาพไม่ชัดอย่างแน่นอน ซึ่งสัญญาณนี้เองที่บอกว่าคุณอาจกำลังประสบกับปัญหาตาแห้ง อันเนื่องจากว่าต่อมไขมันที่เปลือกตาทำงานไม่ปกติ ส่งผลให้ชั้นน้ำตาไม่คงตัว น้ำตาจึงระเหยง่าย และระคายเคืองง่าย มีโอกาสในการติดเชื้อที่เปลือกตาได้ง่าย ซึ่งการสังเกตความผิดปกติของดวงตาและรู้เท่าทันจึงเป็นสิ่งที่ต้องใส่ใจให้ได้มากที่สุด รู้จักกับต่อมไขมันเปลือกตา สำหรับต่อมไขมันเปลือกตานี้คือต่อมไขมันที่อยู่ทั้งเปลือกตาบนและเปลือกตาล่าง โดยมีอยู่เป็นจำนวนมากถึง 70 ต่อม รูเปิดของต่อมไขมันนี้จะอยู่ที่ขอบตา และผลิตไขมันมาสำหรับเคลือบผิวดวงตาเป็นหลัก และเป็นชั้นนอกสุดของน้ำตา ปกติแล้วน้ำตาของมนุษย์มีอยู่ 3 ชั้น […]
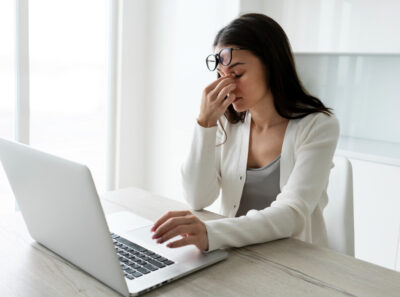
บอกลา 5 ปัญหาสุขภาพที่วัยทำงานต้องเจอ ที่คลินิกเวชศาสตร์ฟื้นฟู รามคำแหง
คลินิกเวชศาสตร์ฟื้นฟู รามคำแหง เป็นอีกหนึ่งผู้ช่วยของวัยทำงานที่กำลังเผชิญกับปัญหาทางสุขภาพอย่างโรคออฟฟิศซินโดรม (Office Syndrome) ที่สามารถเกิดขึ้นได้กับวัยทำงานทุกคน โดยเฉพาะผู้ที่นั่งทำงานในท่าเดิมนาน ๆ จนส่งผลให้เกิดอาการปวดกล้ามเนื้อในส่วนต่าง ๆ และเพื่อป้องกันไม่ให้มีอาการปวดเรื้อรังวันนี้เราจึงมี 5 อาการปวดจากออฟฟิศซินโดรมมาบอกกันว่าเกิดจากอะไรและมีวิธีการรักษาอย่างไร? คลินิกเวชศาสตร์ฟื้นฟู รามคำแหงกับ 5 ปัญหาสุขภาพที่วัยทำงานต้องเจอ คลินิกเวชศาสตร์ฟื้นฟู รามคำแหงกับการรักษาอาการปวดในวัยทำงานด้วยเทคโนโลยีสมัยใหม่ที่ทันสมัย หากใครที่กำลังมีอาการปวดเหล่านี้อยู่ไม่ควรปล่อยไว้จนทำให้ปวดเรื้อรัง […]
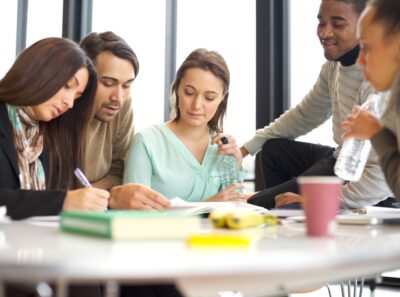
เรียนคอร์สภาษาอังกฤษอย่างไรให้สำเร็จ
ภาษาอังกฤษเป็นภาษาที่จำเป็นสำหรับชีวิตอย่างยิ่ง เพราะไม่ว่าคุณจะไปอยู่ในส่วนใดของโลก คุณก็ไม่สามารถหลีกเลี่ยงภาษาอังกฤษได้แม้แต่วินาทีเดียว โดยเฉพาะอย่างยิ่งในยุคนี้ซึ่งเป็นยุคที่โซเชียลมีเดียกว้างไกล เราจะพบว่าคอร์สภาษาอังกฤษเป็นคอร์สที่ได้รับความนิยมอย่างยิ่ง คนที่ลงเรียนคอร์สสอนภาษาอังกฤษ จะทำอย่างไรให้ได้ผล หรือสำเร็จอย่างรวดเร็ว มาดูพร้อมๆ กัน
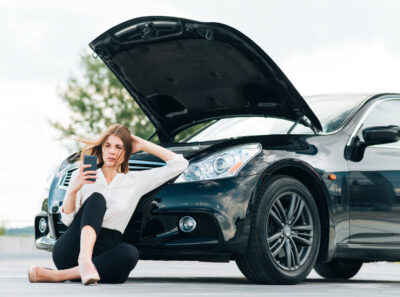
เช็คราคาประกันรถยนต์อย่างไรให้ถูกต้อง
การเช็คราคาประกันรถยนต์เป็นขั้นตอนที่สำคัญในการเลือกซื้อประกันรถยนต์ที่เหมาะสมกับความต้องการและงบประมาณของคุณ ด้วยเหตุนี้ ความถูกต้องในการเช็คราคาประกันรถยนต์จึงเป็นสิ่งที่สำคัญอย่างยิ่ง
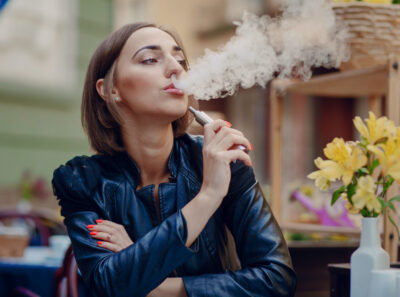
หัว infy หัวพอตไฟฟ้าแบบใส ได้รับความนิยมมากที่สุด
ในบรรดาหัวพอตไฟฟ้า หัว infy จะเป็นหัวพอตรุ่นเดียวเลยก็ว่าได้ ที่ออกแบบมาให้มีหัวพอตเป็นสีใส สามารถมองเห็นน้ำยาด้านในได้อย่างชัดเจน เพราะปกติแล้วหัวพอตไฟฟ้ารุ่นอื่น ๆ มักจะออกแบบให้เป็นสีดำใส ที่เราไม่สามารถมองเห็นสีของน้ำยาได้
หุ้นกู้พลังงาน คืออะไร?
การจัดหาเงินทุนด้านพลังงาน จัดเป็นสิ่งสำคัญไม่แพ้กับการพัฒนาในเรื่องของการจัดหาโซลูชั่นใหม่ ๆ ในการแก้ไขปัญหาเรื่อง พลังงานเลย ซึ่งพันธบัตรพลังงานจัดเป็นเครื่องมือสำคัญในการจัดหาเงินทุน เพื่อนำไปขับเคลื่อนนวัตกรรมทางเทคโนโลยี
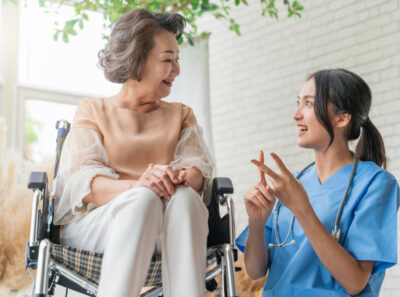
ปัจจัยในการเลือกผู้ให้บริการรับดูแลผู้ป่วย
การเลือกผู้ให้บริการรับดูแลผู้ป่วยเป็นสิ่งสำคัญ เพื่อให้ผู้ป่วยได้รับการดูแลอย่างเหมาะสมและมีคุณภาพชีวิตที่ดี การเลือกผู้ให้บริการรับดูแลผู้ป่วยควรพิจารณาปัจจัยต่างๆ ดังนี้
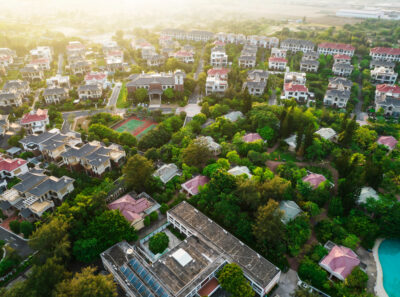
บ้านเดี่ยวบ้านบึง : แนวทางเลือกซื้อบ้านในยุคนี้
บ้านเดี่ยวเป็นที่อยู่อาศัยประเภทหนึ่งที่ได้รับความนิยมอย่างมากในปัจจุบัน ด้วยเหตุผลหลายประการ เช่น มีพื้นที่ใช้สอยส่วนตัวกว้างขวาง เหมาะกับการอยู่อาศัยแบบครอบครัว และความสะดวกสบายในการดูแลรักษา สำหรับใครที่กำลังมองหาบ้านเดี่ยวบ้านบึง เพื่อใช้เป็นที่อยู่อาศัยหรือเพื่อการลงทุน ต่อไปนี้เป็นแนวทางเลือกซื้อบ้านในยุคนี้ที่จะช่วยให้คุณตัดสินใจได้อย่างเหมาะสม 1. กำหนดงบประมาณให้ชัดเจน สิ่งแรกที่ควรทำก่อนเลือกซื้อบ้านคือการกำหนดงบประมาณให้ชัดเจน เพื่อให้คุณสามารถเลือกบ้านที่ตรงกับความต้องการและความสามารถทางการเงินของคุณได้ ในปัจจุบันราคาบ้านเดี่ยวบ้านบึงมีตั้งแต่หลักล้านบาทไปจนถึงหลักสิบล้านบาท ดังนั้นจึงควรพิจารณาปัจจัยต่างๆ เช่น รายได้ ภาระหนี้สิน และความสามารถในการผ่อนชำระ เพื่อกำหนดงบประมาณที่เป็นไปได้ 2. เลือกทำเลที่ตั้งที่เหมาะสม […]
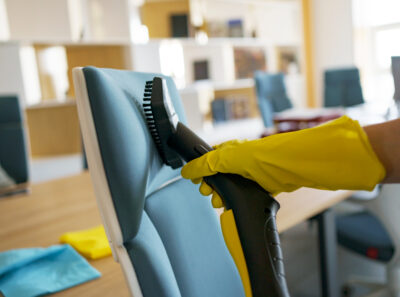
เคล็ดลับทำความสะอาดเก้าอี้สำนักงาน แบบมืออาชีพ
เก้าอี้สำนักงานเป็นเฟอร์นิเจอร์ชิ้นสำคัญที่ต้องใช้งานทุกวัน ด้วยเหตุนี้จึงเป็นเรื่องสำคัญที่จะต้องหมั่นทำความสะอาดเป็นประจำ เพื่อขจัดสิ่งสกปรกและฝุ่นละอองที่สะสมอยู่ ซึ่งอาจก่อให้เกิดปัญหาสุขภาพได้ ต่อไปนี้เป็นเคล็ดลับทำความสะอาดเก้าอี้สำนักงานแบบมืออาชีพ ช่วยให้เก้าอี้สะอาดหมดจด มีอายุการใช้งานยาวนาน และปลอดภัยต่อสุขภาพ 1. เลือกผลิตภัณฑ์ทำความสะอาดที่เหมาะสม การเลือกผลิตภัณฑ์ทำความสะอาดที่เหมาะสมนั้น มีความสำคัญอย่างยิ่ง เพราะผลิตภัณฑ์บางชนิดอาจไม่ปลอดภัยต่อสุขภาพ หรืออาจทำให้เก้าอี้เสียหายได้ ดังนั้น จึงควรพิจารณาปัจจัยต่างๆ ดังนี้ 2. เตรียมอุปกรณ์ให้พร้อม อุปกรณ์ทำความสะอาดเก้าอี้สำนักงาน มีดังนี้ 3. […]